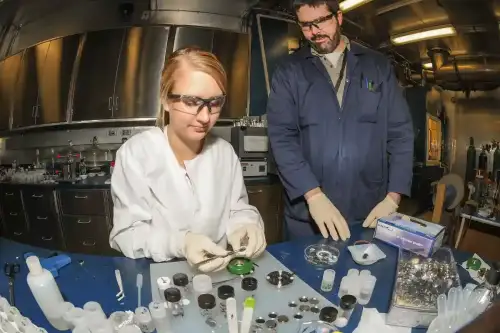
Celiac.com 08/01/2024 - Celiac disease is an autoimmune disorder that disrupts the digestive system, impairing the body's ability to digest and absorb fats. This leads to the excretion of significant amounts of fat in the stool, resulting in symptoms such as diarrhea, abdominal pain, and bloating. Over time, untreated celiac disease can cause severe malnutrition, affect growth and development, and increase the risk of infections and other health complications. Early diagnosis and treatment are crucial to mitigate these effects and improve the quality of life for those affected.
Traditional methods for diagnosing celiac disease include analyzing patient medical history, conducting physical examinations, laboratory tests, and performing intestinal biopsies. However, these methods can be invasive, time-consuming, and sometimes inaccurate due to the subtle and varied nature of celiac disease symptoms. This study explores a novel approach using Raman spectroscopy combined with deep learning models to achieve a rapid, non-invasive, and accurate diagnosis of celiac disease.
Methodology: Plasma Raman Spectroscopy and Deep Learning Models
Celiac.com Sponsor (A12):
Raman spectroscopy is a technique that measures the vibration of molecules in a sample, providing a detailed "biological fingerprint" of its composition. In this study, 50 microliters of plasma samples from 29 celiac disease patients and 30 healthy controls were collected and analyzed using a high-resolution confocal Raman spectrometer. The spectra were obtained by focusing a laser on the sample and measuring the resulting light scattered at different wavelengths. This method captures information about various biomolecules, such as proteins, nucleic acids, carbohydrates, and lipids.
To enhance the diagnostic capability of Raman spectroscopy, the study employed several deep learning models: Convolutional Neural Network (CNN), Multi-Scale Convolutional Neural Network (MCNN), Residual Network (ResNet), and Deep Residual Shrinkage Network (DRSN). These models were trained to differentiate between the Raman spectra of celiac disease patients and healthy individuals. The DRSN model, in particular, showed the highest accuracy, achieving a 95 percent accuracy rate and an area under the curve value of 97.60 percent.
Data Preprocessing and Analysis
The collected Raman spectra were preprocessed to remove background signals and noise using algorithms like airPLS and Smoothing. The data were then split into training and testing sets, with a ratio of 7:3, ensuring that spectra from the same patient were not present in both sets simultaneously. This approach allowed for accurate training and validation of the deep learning models. The study also employed Fourier transformation for low-frequency filtering to enhance the quality of the spectral data.
Results and Discussion
The study found significant differences in the Raman spectra of plasma from celiac disease patients compared to healthy controls. These differences were attributed to variations in the expression of proteins, lipids, and amino acids, which are essential for various biochemical processes. By leveraging these differences, the deep learning models were able to accurately classify the spectra and diagnose celiac disease.
Among the four models tested, the DRSN model outperformed the others in terms of accuracy and noise reduction. Its ability to extract multi-scale features from the spectral data allowed for a more comprehensive analysis and improved diagnostic performance. This model's end-to-end learning approach eliminated the need for manual feature extraction, enhancing automation and reducing the potential for human error.
Conclusion and Significance for Celiac Disease Diagnosis
This study demonstrates that Raman spectroscopy combined with deep learning models, particularly the Deep Residual Shrinkage Network, offers a promising approach for the rapid, non-invasive, and accurate diagnosis of celiac disease. By effectively capturing and analyzing the unique spectral fingerprints of plasma samples, these models can differentiate between celiac disease patients and healthy individuals with high accuracy.
For individuals with celiac disease, this method could lead to earlier diagnosis and treatment, reducing the risk of long-term complications and improving their quality of life. The study's findings also highlight the potential for expanding this diagnostic approach to other diseases, paving the way for more efficient and accurate healthcare solutions. By continuing to refine and validate these techniques, researchers can contribute to the development of better diagnostic tools and improved patient outcomes.
Read more at: nature.com
Recommended Comments
There are no comments to display.
Create an account or sign in to comment
You need to be a member in order to leave a comment
Create an account
Sign up for a new account in our community. It's easy!
Register a new accountSign in
Already have an account? Sign in here.
Sign In Now